ML-Powered Document Understanding Solution
We didn't just tweak the approach to document understanding—we reimagined it. Our ML solution went beyond OCR with advanced features.
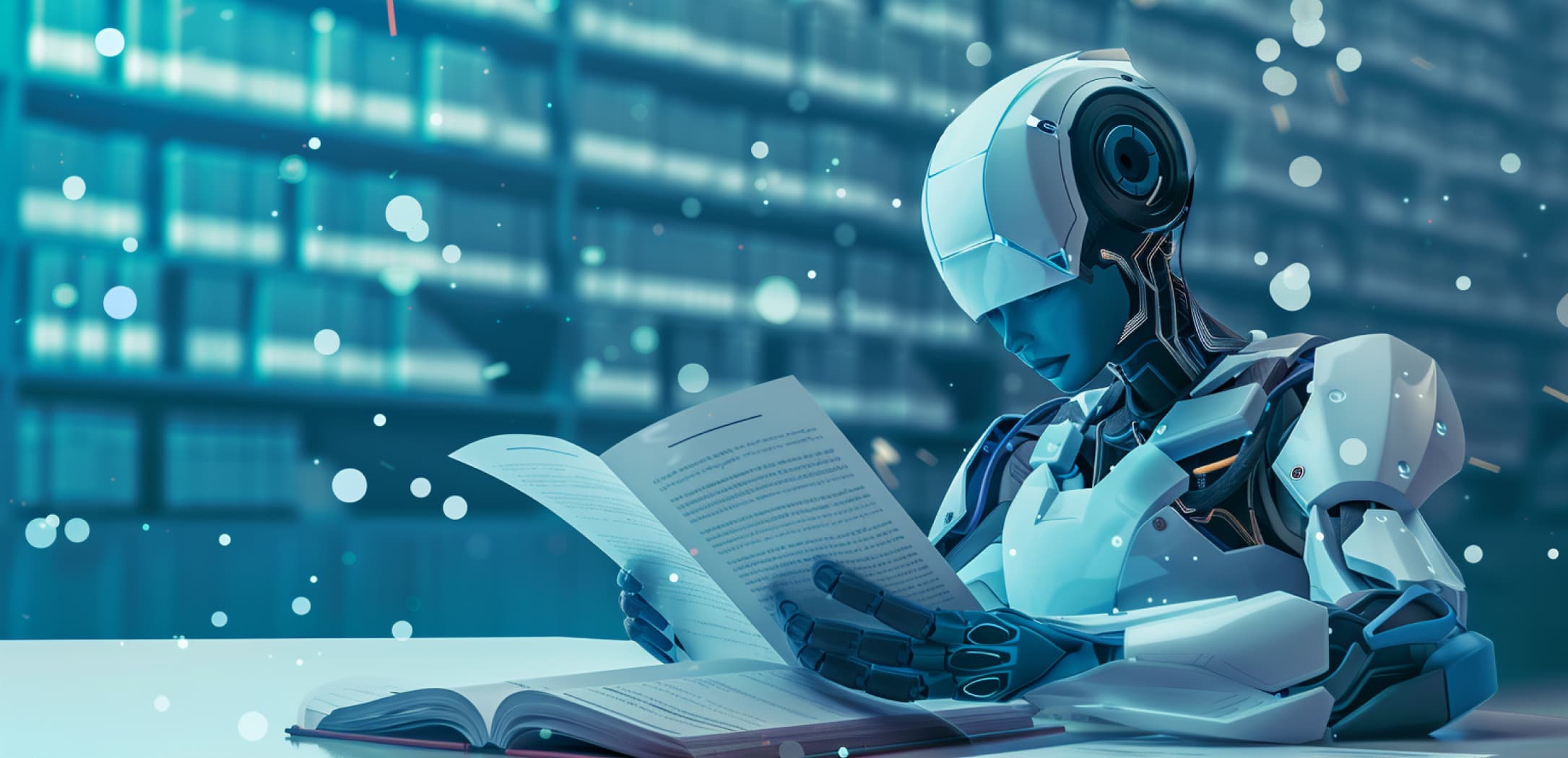
Story behind
Fintech platforms adopt AI not because it's trendy, but because it genuinely improves the user experience. Our client Flexidea recognized that traditional manual data entry is not only hard work but also prone to mistakes. Invoices are complex and require careful handling of various details like invoice numbers, company names, dates, and account numbers. This prompted our machine learning (ML) engineers to develop an efficient auto-fill model for invoices.
Example
Extract desired text fields from photos and scans of unstructured invoices in various languages.


Goal
Flexidea's objective was to create a solution for auto-filling invoice details from documents uploaded to their servers. The solution needed to process invoices in various formats and handle multiple languages, primarily Latvian and English. Here’s what they had:
- 7498 PDFs: 5343 readable, 2155 non-readable or image-wrapped PDFs
- 1938 Images: JPEG, PNG, BMP, and TIFF formats
- 168 other types: XLS/XLSX, e-doc, DOC/DOCX, etc.
Out of the total 9604 documents, nearly half (4093) required data extraction due to being image-based or poorly scanned. The system had to focus on extracting invoice information from raw texts and setting up a tool to extract texts from images and non-readable PDFs.
Challenges
Normally businesses would try to solve a task like this with Optical Character Recognition (OCR). Most available solutions for data extraction are OCR-based.
However, while OCR can extract text, it’s unable to understand the structure or categorize fields like invoice numbers or dates. This leads to inaccuracies and constant manual corrections. OCR also struggles with multi-language documents and poor-quality images.
This is why we decided to take the document understanding approach rather than mere text recognition. Still, this path posed its own challenges:
System performance
Dependent on the quality of existing language models. Future improvements may require training larger models.
Inference speed
Varies based on document size, model architecture, and infrastructure. Real-time speed isn't guaranteed but should be within a few minutes per document.
Multiple languages
Handling documents with multiple languages can reduce model performance.
Document quality
Blurry or poorly lit photos, or damaged invoices, may impact performance..
.jpg)
Tensorway’s solution
We didn't just tweak the approach to document understanding—we reimagined it. Our ML solution went beyond OCR with advanced features:
Grasping invoice structure
Using Named Entity Recognition (NER), our model pinpointed specific data fields like invoice numbers and company names with high accuracy.
Mastering Latvian
We crafted a specialized NER model for Latvian invoices, tackling the nuances of grammar and vocabulary head-on.
Ready for more languages
Our solution is built to scale, allowing Flexidea to expand its reach internationally.
Cutting errors,
boosting speed
Automation drastically reduced human errors and sped up processing.
By incorporating feedback from both model outputs and user modifications, we refined Flexidea’s invoice auto-filling model. We addressed complexities like handling documents in multiple languages or processing poor-quality inputs, enhancing our system's performance.
Highlight: The Latvian language model
One of the standout achievements was the development of a specialized model for the Latvian language. While AI models for widely spoken languages are prevalent:
We identified a gap in the market for Latvian linguistic processing.
Developing a model for such a specific language required intensive data training and localization efforts.
Our success in this area exemplifies our commitment to addressing diverse market needs, even niche ones like invoice financing software.
As a result...
Our solution doesn't just crunch data; it saves the time and effort required when manually filling invoices. Flexidea's employees can now focus on higher-value tasks, creating a more efficient and productive work environment. Improved accuracy means smoother client experiences and a stronger reputation for reliability.
With this approach, Flexidea can look forward to further innovations, reaching more markets, and continuously simplifying financial processes for clients globally.
Effortless data input
Users simply upload any invoice format, and our AI handles the rest, slashing manual effort and processing time.
Precision data identification
Tensorway’s advanced document understanding ensures accurate extraction of crucial fields, even in complex layouts or Latvian documents.
Efficiency & accuracy
Automation cuts down errors and operational costs, leading to faster processing times.
Project team, steps, and timeline
Team
Extracting raw texts & creating training data
Tuning and training the NER model
Improving the NER model and selecting the best architecture
Building & deploying service
Other possible
applications
Tensorway’s document understanding solution isn't just for fintech; it has a broad range of applications across industries.
Healthcare
Streamline the management of patient records, insurance claims, and billing
Legal sector
Automate the review and categorization of contracts, legal documents, and case files.
Education institutions
Handle student records, admissions documents, and administrative paperwork more efficiently.
Nonprofits
Automate the processing of donations, grants, and expense reports, ensuring accurate record-keeping and freeing up valuable time for mission-critical tasks.
Retail and eCommerce
Simplify the management of invoices, purchase orders, and shipping documents.